DOI |
Paper Title |
10.21058/gjecs.2021.61001 |
Performance Prediction of SiOC on Insulator based PICs |
Author Name |
Volume No., Issue No., Year, & Page No. |
Ameet Kumar, Abi Waqas, Faisal Memon, Umair Ahmed Korai |
Vol. 6, No. 1, March 2021, pp. 1-10 |
Abstract:
In this emerging world of Photonics Silicon oxycarbide (SiOC) is introduced as a platform that has a wide range of tunable refractive indexes that possess very
low absorption coefficients. Its physical properties likewise (Optical) and chemical properties can be altered over a large scale in different applications
through its composition. In this manuscript, the results obtained using waveguides and directional couplers by multiple simulations that relied on the SiOC
technology. In this paper, most simplified design of the coupling coefficient in a certain defined range of width, gap as well as coupling length is proposed.
The directional coupler and the waveguide building block’s mathematical models are parameterized. In our defined model, the passive devices will be exploited in
available circuit simulators used commercially for the stochastic and circuit simulations scheme for SiOC based photonic circuits.
|
Keywords:Photonics, SiOC, Circuit Simulation, Building Blocks, Stochastic Analysis
|
Full Text: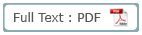 |
References:
- Avgerou, M. Smit et al., ‘An introduction to InP-based generic integration technology’, Semicond. Sci. Technol., vol. 29, no. 8, p. 083001, Jun. 2014.
- D. Melati et al., ‘Validation of the Building-Block-Based Approach for the Design of Photonic Integrated Circuits’, J. Light. Technol., vol. 30, no. 23, pp. 3610–3616, Dec. 2012.
- M. Hochberg and T. Baehr-Jones, ‘Towards fabless silicon photonics’, Nat. Photonics, vol. 4, no. 8, pp. 492–494, Aug. 2010.
- T. Korthorst, R. Stoffer, and A. Bakker, ‘Photonic IC design software and process design kits’, Adv. Opt. Technol., vol. 4, no. 2, Jan. 2015.
- X. J. M. Leijtens, P. Le Lourec, and M. K. Smit, ‘S-matrix oriented CAD-tool for simulating complex integrated optical circuits’, IEEE J. Sel. Top. Quantum Electron., vol. 2, no. 2, pp. 257–262, Jun. 1996.
- L. David, R. Bhandavat, U. Barrera, and G. Singh, ‘Silicon oxycarbide glass-graphene composite paper electrode for long-cycle lithium-ion batteries’, Nat. Commun., vol. 7, no. 1, p. 10998, Apr. 2016.
- V. S. Pradeep, M. Graczyk-Zajac, R. Riedel, and G. D. Soraru, ‘New Insights in to the Lithium Storage Mechanism in Polymer Derived SiOC Anode Materials’, Electrochim. Acta, vol. 119, pp. 78–85, Feb. 2014.
- A. Grill, ‘Plasma enhanced chemical vapor deposited SiCOH dielectrics: from low- k to extreme low- k
- H. J. Kim, Q. Shao, and Y.-H. Kim, ‘Characterization of low-dielectric-constant SiOC thin films deposited by PECVD for interlayer dielectrics of multilevel interconnection’, Surf. Coatings Technol., vol. 171, no. 1–3, pp. 39–45, Jul. 2003.
- M. R. Wang, Rusli, J. L. Xie, N. Babu, C. Y. Li, and K. Rakesh, ‘Study of oxygen influences on carbon doped silicon oxide low k thin films deposited by plasma enhanced chemical vapor deposition’, J. Appl. Phys., vol. 96, no. 1, pp. 829–834, Jul. 2004.
- V. Nikas et al., ‘The origin of white luminescence from silicon oxycarbide thin films’, Appl. Phys. Lett., vol. 104, no. 6, p. 061906, Feb. 2014.
- A. Karakuscu, R. Guider, L. Pavesi, and G. D. SorarÃ1, ‘White Luminescence from Solar Gel-Derived SiOC Thin Films’, J. Am. Ceram. Soc., vol. 92, no. 12, pp. 2969–2974, Dec. 2009.
- Y. PENG, J. ZHOU, X. ZHENG, B. ZHAO, and X. TAN, ‘STRUCTURE AND PHOTOLUMINESCENCE PROPERTIES OF SILICON OXYCARBIDE THIN FILMS DEPOSITED BY THE RF REACTIVE SPUTTERING’, Int. J. Mod. Phys. B, vol. 25, no. 22, pp. 2983–2990, Sep. 2011.
- Y. Ding, H. Shirai, and D. He, ‘White light emission and electrical properties of silicon oxycarbide-based metal–oxide–semiconductor diode’, Thin Solid Films, vol. 519, no. 8, pp. 2513–2515, Feb. 2011.
- G. Bellocchi, G. Franzò, M. Miritello, and F. Iacona, ‘White light emission from Eu-doped SiOC films’.
- Waqas, A., Memon, F. A., & Korai, U. A. (2020). Experimental validation of a building block of passive devices and stochastic analysis of PICs based on SiOC technology. Optics Express, 28(15), 21420-21431.
- A. Waqas, D. Melati, and A. Melloni, ‘Sensitivity Analysis and Uncertainty Mitigation of Photonic Integrated Circuits’, J. Light. Technol., vol. 35, no. 17, pp. 3713–3721, Sep. 2017.
- D. Melati, A. Waqas, and A. Melloni, ‘Stocastic photonics: Tools and approaches for the analysis and optimization of integrated circuits’, in 2017 Opto-Electronics and Communications Conference (OECC) and Photonics Global Conference (PGC), 2017, pp. 1–3.
- A. Waqas, D. Melati, and A. Melloni, ‘Stochastic simulation and sensitivity analysis of photonic circuit through Morris and Sobol method’, in Optical Fiber Communication Conference, 2017, p. Th2A.3.
- X. Chen, M. Mohamed, Z. Li, L. Shang, and A. R. Mickelson, ‘Process variation in silicon photonic devices’, Appl. Opt., vol. 52, no. 31, p. 7638, Nov. 2013.
- A. Waqas, D. Melati, B. S. Chowdhry, and A. Melloni, ‘Efficient Variability Analysis of Photonic Circuits by Stochastic Parametric Building Blocks’, IEEE J. Sel. Top. Quantum Electron., vol. 26, no. 2, pp. 1–8, Mar. 2020.
- A. Waqas, D. Melati, P. Manfredi, and A. Melloni, ‘Stochastic process design kits for photonic circuits based on polynomial chaos augmented macro-modelling’, Opt. Express, vol. 26, no. 5, p. 5894, Mar. 2018.
|
DOI |
Paper Title |
10.21058/gjecs.2021.61002 |
Rail Surface Faults Identification from Low Quality Image Data Using Machine Learning Algorithms |
Author Name |
Volume No., Issue No., Year, & Page No. |
Asfar Arain, Tanweer Hussain, Sanaullah Mehran Ujjan, Bhawani Shankar Chowdhry, Tariq Rafique Memon |
Vol. 6, No. 1, March 2021, pp. 11-21 |
Abstract:
Rail surface faults or deformities that form on railhead of the track, owe their existence to various operational and environmental factors.
To ensure comfortable and safe operation of railway vehicles, on-time detection of these surface faults is necessary. It is also of paramount
importance that fault types are identified because it can lead to the identification of causes. This eventually leads to development of better
maintenance strategies. Automation of the rail inspection is highly desirable because it results in accurate, robust, and cost-effective condition
monitoring of the railway track. Automated systems of track monitoring currently in use are highly sophisticated instrumentation systems, with
high-speed cameras and equipped with state-of-the-art level hardware. In this research, a preliminary work towards developing a low-cost rail
condition monitoring system is presented. A suitable action camera EKEN-H9R is used to acquire videos of track surface. This data is preprocessed and
later used to train data-driven models for fault identification. A comparative analysis of multiple data-driven classification algorithms is
conducted on the acquired data and
research is concluded with support vector machine algorithm which was able to achieve about 96% accuracy on the fault classification task.
|
Keywords:Rail Surface faults, Fault identification, Condition monitoring, Machine learning, Data-driven models, Classification, Noisy data.
|
Full Text: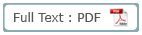 |
References:
- A. Jamshidi et al., “A Big Data Analysis Approach for Rail Failure Risk Assessment,” Risk Anal., vol. 37, no. 8, pp. 1495–1507, 2017.
- Q. Y. Li, Z. D. Zhong, M. Liu, and W. W. Fang, Smart Railway Based on the Internet of Things. Elsevier Inc., 2017.
- S. Faghih-Roohi, S. Hajizadeh, A. Nunez, R. Babuska, and B. De Schutter, “Deep convolutional neural networks for detection of rail surface defects,” Proc. Int. Jt. Conf. Neural Networks, vol. 2016-Octob, pp. 2584–2589, 2016.
- S. Alahakoon, Y. Q. Sun, M. Spiryagin, and C. Cole, “Rail Flaw Detection Technologies for Safer, Reliable Transportation: A Review,” J. Dyn. Syst. Meas. Control. Trans. ASME, vol. 140, no. 2, 2018.
- N. Alnaimi and U. Qidwai, “IoT Based on-the-fly Visual Defect Detection in Railway Tracks,” 2020 IEEE Int. Conf. Informatics, IoT, Enabling Technol. ICIoT 2020, pp. 627–631, 2020.
- X. Wei, D. Wei, D. Suo, L. Jia, and Y. Li, “Multi-target defect identification for railway track line based on image processing and improved YOLOv3 model,” IEEE Access, vol. 8, pp. 61973–61988, 2020.
- M. Niu, K. Song, L. Huang, qi wang, Y. Yan, and Q. Meng, “Unsupervised Saliency Detection of Rail Surface Defects using Stereoscopic Images,” IEEE Trans. Ind. Informatics, vol. 3203, no. c, pp. 1–1, 2020.
- J. Gan, J. Wang, H. Yu, Q. Li, and Z. Shi, “Online Rail Surface Inspection Utilizing Spatial Consistency and Continuity,” IEEE Trans. Syst. Man, Cybern. Syst., vol. 50, no. 7, pp. 2741–2751, 2020.
- J. Wang, Q. Li, J. Gan, H. Yu, and X. Yang, “Surface defect detection via entity sparsity pursuit with intrinsic priors,” IEEE Trans. Ind. Informatics, vol. 16, no. 1, pp. 141–150, 2020.
- A. A. Shah, B. S. Chowdhry, T. D. Memon, I. H. Kalwar, and J. Andrew Ware, “Real time identification of railway track surface faults using canny edge detector and 2D discrete wavelet transform,” Ann. Emerg. Technol. Comput., vol. 4, no. 2, pp. 53–60, 2020.
- H. Yu et al., “A Coarse-to-Fine Model for Rail Surface Defect Detection,” IEEE Trans. Instrum. Meas., vol. 68, no. 3, pp. 656–666, 2019.
- Y. Min, B. Xiao, J. Dang, B. Yue, and T. Cheng, “Real time detection system for rail surface defects based on machine vision,” Eurasip J. Image Video Process., vol. 2018, no. 1, pp. 1–11, 2018.
- L. Zhuang, L. Wang, Z. Zhang, and K. L. Tsui, “Automated vision inspection of rail surface cracks: A double-layer data-driven framework,” Transp. Res. Part C Emerg. Technol., vol. 92, no. May, pp. 258–277, 2018.
- Q. Li et al., “A cyber-enabled visual inspection system for rail corrugation,” Futur. Gener. Comput. Syst., vol. 79, pp. 374–382, 2018.
- J. Gan, Q. Li, J. Wang, and H. Yu, “A Hierarchical Extractor-Based Visual Rail Surface Inspection System,” IEEE Sens. J., vol. 17, no. 23, pp. 7935–7944, 2017.
- K. Ma, T. F. Y. Vicente, D. Samaras, M. Petrucci, and D. L. Magnus, “Texture classification for rail surface condition evaluation,” 2016 IEEE Winter Conf. Appl. Comput. Vision, WACV 2016, 2016.
- J. Gan, J. Wang, H. Yu, Q. Li, and Z. Shi, “Online Rail Surface Inspection Utilizing Spatial Consistency and Continuity,” IEEE Trans. Syst. Man, Cybern. Syst., vol. 50, no. 7, pp. 2741–2751, 2020.
- Y. W. Lin, C. C. Hsieh, W. H. Huang, S. L. Hsieh, and W. H. Hung, “Railway Track Fasteners Fault Detection using Deep Learning,” 2019 IEEE Eurasia Conf. IOT, Commun. Eng. ECICE 2019, no. October, pp. 187–190, 2019.
- K. Shaikh, S. M. Ujjan, I. H. Kalwar, and B. S. Chowdhry, “Indirect identification of varying conicity levels of wheel tread using convolutional neural networks,” Int. J. Adv. Sci. Technol., vol. 29, no. 7, pp. 2537–2547, 2020.
- W. He, Y. He, B. Li, and C. Zhang, “A Naive-Bayes-Based Fault Diagnosis Approach for Analog Circuit by Using Image-Oriented Feature Extraction and Selection Technique,” IEEE Access, vol. 8, pp. 5065–5079, 2020.
|
DOI |
Paper Title |
10.21058/gjecs.2021.62001 |
Railway Track Fault Analysis using Dynamic ROI Detection in Cluttered Environment using Deep Learning |
Author Name |
Volume No., Issue No., Year, & Page No. |
Ghulam Hyder Palli, Khuhed Memon, Bhawani Shankar Chowdhry, Azam Rafique Memon, Tanweer Hussain
|
Vol. 6, No. 2, September 2021, pp. 1-15 |
Abstract:
The safety of the railway track can be accomplished by the regular inspection of the track by detecting the faults which occur due to several parameters. The fault analysis is very necessary, if they are not timely monitored then the train can face severe accidents which may result in the loss of several lives. The condition monitoring of the track is taken manually these days and the world is moving towards automation to avoid human error and acquire high accuracy. The inspection of faults can be carried out by either using sensors or image processing techniques in which Deep Learning can play a vital role to have efficient systems. This research is carried out using the Deep Learning techniques, the rail surface faults such as squats and corrugation are monitored by the Deep Learning techniques with an addition of IOT (Internet of Things) feature for real time fault alerts with GPS locations on Desktop Systems using the Web Portal.
|
Keywords:Railway Fault Analysis, Dynamic ROI, Cluttered Environment, Deep Learning, IOT.
|
Full Text: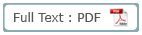 |
References:
- Istiar and H. Widyastuti, “Review study of irregularity track monitoring based on inertial measure unit and power spectral density analysis,” IOP Conference Series: Materials Science and Engineering, vol. 650, no. 1, 2019, doi: 10.1088/1757-899X/650/1/012046.
- A. Falamarzi, S. Moridpour, and M. Nazem, “A review on existing sensors and devices for inspecting railway infrastructure,” Jurnal Kejuruteraan, vol. 31, no. 1, pp. 1–10, 2019.
- T. Wang, F. Yang, and K. L. Tsui, “Real-time detection of railway track component via one-stage deep learning networks,” Sensors (Switzerland), vol. 20, no. 15, pp. 1–15, 2020, doi: 10.3390/s20154325.
- X. Wei, Z. Yang, Y. Liu, D. Wei, L. Jia, and Y. Li, “Railway track fastener defect detection based on image processing and deep learning techniques: A comparative study,” Engineering Applications of Artificial Intelligence, vol. 80, no. September 2018, pp. 66–81, 2019, doi: 10.1016/j.engappai.2019.01.008.
- L. Li, R. Sun, S. Zhao, X. Chai, S. Zheng, and R. Shen, “Semantic-Segmentation-Based Rail Fastener State Recognition Algorithm,” Mathematical Problems in Engineering, vol. 2021, 2021, doi: 10.1155/2021/8956164.
- T. Prasongpongchai, T. H. Chalidabhongse, and S. Leelhapantu, “A vision-based method for the detection of missing rail fasteners,” Proceedings of the 2017 IEEE International Conference on Signal and Image Processing Applications, ICSIPA 2017, pp. 419–424, 2017, doi: 10.1109/ICSIPA.2017.8120647.
- X. Gibert, V. M. Patel, and R. Chellappa, “Robust fastener detection for autonomous visual railway track inspection,” Proceedings - 2015 IEEE Winter Conference on Applications of Computer Vision, WACV 2015, pp. 694–701, 2015, doi: 10.1109/WACV.2015.98.
- H. Feng, Z. Jiang, F. Xie, P. Yang, J. Shi, and L. Chen, “Automatic fastener classification and defect detection in vision-based railway inspection systems,” IEEE Transactions on Instrumentation and Measurement, vol. 63, no. 4, pp. 877–888, 2014, doi: 10.1109/TIM.2013.2283741.
- J. Yang, W. Tao, M. Liu, Y. Zhang, H. Zhang, and H. Zhao, “An efficient direction field-based method for the detection of fasteners on high-speed railways,” Sensors, vol. 11, no. 8, pp. 7364–7381, 2011, doi: 10.3390/s110807364.
- X. Yuan, B. Liu, and H. Chen, “Algorithm and program design for fastener locating and detection using wavelet transformation and template matching,” International Conference on Communication Technology Proceedings, ICCT, vol. 2017-Octob, no. c, pp. 1116–1121, 2018, doi: 10.1109/ICCT.2017.8359808.
- M. Kavya, T. K. Sen, V. Vaisakh, and G. Renjini, “Solar Based Railway Track Fault Detection System,” vol. 9, no. 1, pp. 1–6, 2020.
- B. Chowdhury, B. Das, and A. Ghosh, “Railway Track Crack Detection Technique,” Ijireeice, vol. 6, no. 10, pp. 37–37, 2018, doi: 10.17148/ijireeice.2018.6106.
- P. Lad and M. Pawar, “Evolution of railway track crack detection system,” 2016 2nd IEEE International Symposium on Robotics and Manufacturing Automation, ROMA 2016, 2017, doi: 10.1109/ROMA.2016.7847816.
- N. Alnaimi and U. Qidwai, “IoT Based on-the-fly Visual Defect Detection in Railway Tracks,” 2020 IEEE International Conference on Informatics, IoT, and Enabling Technologies, ICIoT 2020, pp. 627–631, 2020, doi: 10.1109/ICIoT48696.2020.9089560.
- X. Wei, D. Wei, D. Suo, L. Jia, and Y. Li, “Multi-target defect identification for railway track line based on image processing and improved YOLOv3 model,” IEEE Access, vol. 8, pp. 61973–61988, 2020, doi: 10.1109/ACCESS.2020.2984264.
- A. Jamshidi et al., “A Big Data Analysis Approach for Rail Failure Risk Assessment,” Risk Analysis, vol. 37, no. 8, pp. 1495–1507, 2017, doi: 10.1111/risa.12836.
- A. A. Shah, B. S. Chowdhry, T. D. Memon, I. H. Kalwar, and J. Andrew Ware, “Real time identification of railway track surface faults using canny edge detector and 2D discrete wavelet transform,” Annals of Emerging Technologies in Computing, vol. 4, no. 2, pp. 53–60, 2020, doi: 10.33166/AETiC.2020.02.005.
- Y. Min, B. Xiao, J. Dang, B. Yue, and T. Cheng, “Real time detection system for rail surface defects based on machine vision,” Eurasip Journal on Image and Video Processing, vol. 2018, no. 1, pp. 1–11, 2018, doi: 10.1186/s13640-017-0241-y.
- “Jetson Nano vs Raspberry Pi. Nvidia recently announced about its new… | by Aniket Kumar | Analytics Vidhya | Medium.” https://medium.com/analytics-vidhya/jetson-nano-vs-raspberry-pi-e84642555adc (accessed Nov. 07, 2021).
- “NVIDIA Jetson Nano Developer Kit | NVIDIA Developer.” https://developer.nvidia.com/embedded/jetson-nano-developer-kit (accessed Nov. 07, 2021).
- “New Jetson Nano 2GB Developer Kit | NVIDIA.” https://www.nvidia.com/en-us/autonomous-machines/embedded-systems/jetson-nano-developer-kit/ (accessed Nov. 07, 2021).
- “Original EKEN H9R Action Camera | Waterproof action camera, 4k video camera, best budget action camera, Sports cam, helmet camera | EKEN Official Website.” https://www.eken.com/H9R (accessed Nov. 07, 2021).
- “Eken V8s vs Eken H9R vs EKEN H6S Comparison.” https://www.findpare.com/comparison/eken-v8s-VS-eken-h9r-VS-eken-h6s (accessed Nov. 07, 2021).
- “In-Depth: Interface ublox NEO-6M GPS Module with Arduino.” https://lastminuteengineers.com/neo6m-gps-arduino-tutorial/ (accessed Nov. 07, 2021).
- “Keras Applications.” https://keras.io/api/applications/ (accessed Nov. 07, 2021).
|
DOI |
Paper Title |
10.21058/gjecs.2021.62002 |
INDUCTION MOTOR FAULT DETECTION AND CLASSIFICATION USING THERMAL IMAGES AND DEEP LEARNING |
Author Name |
Volume No., Issue No., Year, & Page No. |
Saira Parveen, Tanweer Hussain, Dileep Kumar, and Bhawani Shankar Chowdhry
|
Vol. 6, No. 2, September 2021, pp. 16-31 |
Abstract:
Electro-mechanical systems such as induction motors (IMs) are widely used in industry as driving forces for multiple small and large-scale systems. The advantages of IMs like high reliability, high efficiency, and robust operation have resulted in their widespread applications. The operation of the motors under various electrical and mechanical variations degrades their performance and reduces their life span. For the safe and sustained operation of IMs, existing condition monitoring methods use different sensors for diagnosis. Still, these methods pose some difficulties, such as they require proper sensor installation and are prone to noise. Therefore, more effective diagnosis methods are needed for effective condition monitoring of machines. Infrared thermography (IRT) as a non-invasive technique can efficiently be used to address sensor-related problems. Recently, IRT as a noncontact technique has been widely adopted for condition monitoring of rotating machines. In this work, IRT-based three-phase motor fault detection and identification is proposed. The thermal images acquired under different motor operating conditions are fed to the deep learning (DL) models for fault identification. The supervised DL methods effectively classified the motor conditions owing to their high generalization capabilities. The obtained results demonstrate that the RESNET-50 model achieved the best classification results with an accuracy of 97% without any manual feature extraction method.
|
Keywords:Induction motor; Thermal imaging; Deep learning; Condition monitoring; Fault diagnosis.
|
Full Text: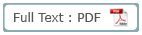 |
References:
- Y. Lei, B. Yang, X. Jiang, F. Jia, N. Li, and A. K. Nandi, "Applications of machine learning to machine fault diagnosis: A review and roadmap," Mechanical Systems and Signal Processing, vol. 138, p. 106587, 2020.
- D. K. Soother and J. Daudpoto, "A brief review of condition monitoring techniques for the induction motor," Transactions of the Canadian Society for Mechanical Engineering, vol. 43, pp. 499-508, 2019.
- D. K. Soother, J. Daudpoto, and A. Shaikh, "Vibration Measurement System for the Low Power Induction Motor," Engineering Science And Technology International Research Journal, vol. 2, pp. 53-57, 2018.
- M. Hussain, D. K. Soother, I. H. Kalwar, T. D. Memon, Z. A. Memon, K. Nisar, et al., "Stator Winding Fault Detection and Classification in Three-Phase Induction Motor," Intelligent Automation And Soft Computing, vol. 29, pp. 869-883, 2021.
- L. Frosini, "Novel Diagnostic Techniques for Rotating Electrical Machines—A Review," Energies, vol. 13, p. 5066, 2020.
- A. Choudhary, D. Goyal, S. L. Shimi, and A. Akula, "Condition monitoring and fault diagnosis of induction motors: A review," Archives of Computational Methods in Engineering, vol. 26, pp. 1221-1238, 2019.
- D. A. Nicola, M. A. Rosen, C. A. Bulucea, and D. C. Cismaru, "Some aspects of sustainable energy conversion in electric railway vehicles with traction induction motors," in Recent Advances in Systems Science: Proc. 17th Int. Conf. on Systems, 2013, pp. 16-19.
- C. A. Bulucea, D. A. Nicola, M. A. Rosen, N. E. Mastorakis, and C. A. Bulucea, "Operation analysis of AC traction motors in terms of electromagnetic torque capability on sustainable railway vehicles," in MATEC Web of Conferences, 2016, p. 02005.
- G. Singh, C. A. Kumar, and V. Naikan, "Effectiveness of Current Envelope analysis to detect broken rotor bar and inter turn faults in an inverter fed induction motor drive," in 2015 international conference on power and advanced control engineering (ICPACE), 2015, pp. 191-194.
- A. Choudhary, S. Shimi, and A. Akula, "Bearing Fault Diagnosis of Induction Motor Using Thermal Imaging," in 2018 International Conference on Computing, Power and Communication Technologies (GUCON), 2018, pp. 950-955.
- Z. Jia, Z. Liu, C.-M. Vong, and M. Pecht, "A rotating machinery fault diagnosis method based on feature learning of thermal images," IEEE Access, vol. 7, pp. 12348-12359, 2019.
- G. Devarajan, M. Chinnusamy, and L. Kaliappan, "Detection and classification of mechanical faults of three phase induction motor via pixels analysis of thermal image and adaptive neuro-fuzzy inference system," Journal of Ambient Intelligence and Humanized Computing, vol. 12, pp. 4619-4630, 2021.
- W. Zhang, G. Peng, C. Li, Y. Chen, and Z. Zhang, "A new deep learning model for fault diagnosis with good anti-noise and domain adaptation ability on raw vibration signals," Sensors, vol. 17, p. 425, 2017.
- A. Glowacz and Z. Glowacz, "Diagnosis of the three-phase induction motor using thermal imaging," Infrared physics & technology, vol. 81, pp. 7-16, 2017.
- L. Yongbo, D. Xiaoqiang, W. Fangyi, W. Xianzhi, and Y. Huangchao, "Rotating machinery fault diagnosis based on convolutional neural network and infrared thermal imaging," Chinese Journal of Aeronautics, vol. 33, pp. 427-438, 2020.
- D. K. Soother, J. Daudpoto, N. R. Harris, M. Hussain, S. Mehran, I. H. Kalwar, et al., "The Importance of Feature Processing in Deep-Learning-Based Condition Monitoring of Motors," Mathematical Problems in Engineering, vol. 2021, 2021.
- S. M. Ujjan, I. H. Kalwar, B. S. Chowdhry, T. D. Memon, and D. K. Soother, "Adhesion level identification in wheel-rail contact using deep neural networks," 3C Technologia, vol. Special issue, pp. 217-231, 2020.
- G. Singh and V. Naikan, "Infrared thermography based diagnosis of inter-turn fault and cooling system failure in three phase induction motor," Infrared Physics & Technology, vol. 87, pp. 134-138, 2017.
- Y. Li, X. Wang, S. Si, and X. Du, "A new intelligent fault diagnosis method of rotating machinery under varying-speed conditions using infrared thermography," Complexity, vol. 2019, 2019.
- Y. L. dit Leksir, M. Mansour, and A. Moussaoui, "Localisation of thermal anomalies in electrical equipment using Infrared Thermography and support vector machine," Infrared Physics & Technology, vol. 89, pp. 120-128, 2018.
- E. Resendiz-Ochoa, R. A. Osornio-Rios, J. P. Benitez-Rangel, R. D. J. Romero-Troncoso, and L. A. Morales-Hernandez, "Induction motor failure analysis: An automatic methodology based on infrared imaging," IEEE Access, vol. 6, pp. 76993-77003, 2018.
- A. K. Al-Musawi, F. Anayi, and M. Packianather, "Three-phase induction motor fault detection based on thermal image segmentation," Infrared Physics & Technology, vol. 104, p. 103140, 2020.
- M. Khanjani and M. Ezoji, "Electrical fault detection in three-phase induction motor using deep network-based features of thermograms," Measurement, vol. 173, p. 108622, 2021.
- M. B. Najafi, Yasser; Mirimani, and Seyyed Mehdi, "Thermal image of equipment (Induction Motor)," 2020.
- K. Simonyan and A. Zisserman, "Very deep convolutional networks for large-scale image recognition," arXiv preprint arXiv:1409.1556, 2014.
- Z. Hameed, S. Zahia, B. Garcia-Zapirain, J. Javier Aguirre, and A. María Vanegas, "Breast cancer histopathology image classification using an ensemble of deep learning models," Sensors, vol. 20, p. 4373, 2020.
- K. He, X. Zhang, S. Ren, and J. Sun, "Deep residual learning for image recognition," in Proceedings of the IEEE conference on computer vision and pattern recognition, 2016, pp. 770-778.
- Y. Tian, Y. Wang, D. Krishnan, J. B. Tenenbaum, and P. Isola, "Rethinking few-shot image classification: a good embedding is all you need?," in Computer Vision–ECCV 2020: 16th European Conference, Glasgow, UK, August 23–28, 2020, Proceedings, Part XIV 16, 2020, pp. 266-282.
- D. K. Soother, I. H. Kalwar, T. Hussain, B. S. Chowdhry, S. M. Ujjan, and T. D. Memon, "A Novel Method Based on UNET for Bearing Fault Diagnosis," Computers Materials and Continua, vol. 69, pp. 393-408, 2021.
|